Career Trends: December 17, 2022
Curated by the Knowledge Team of ICS Career GPS
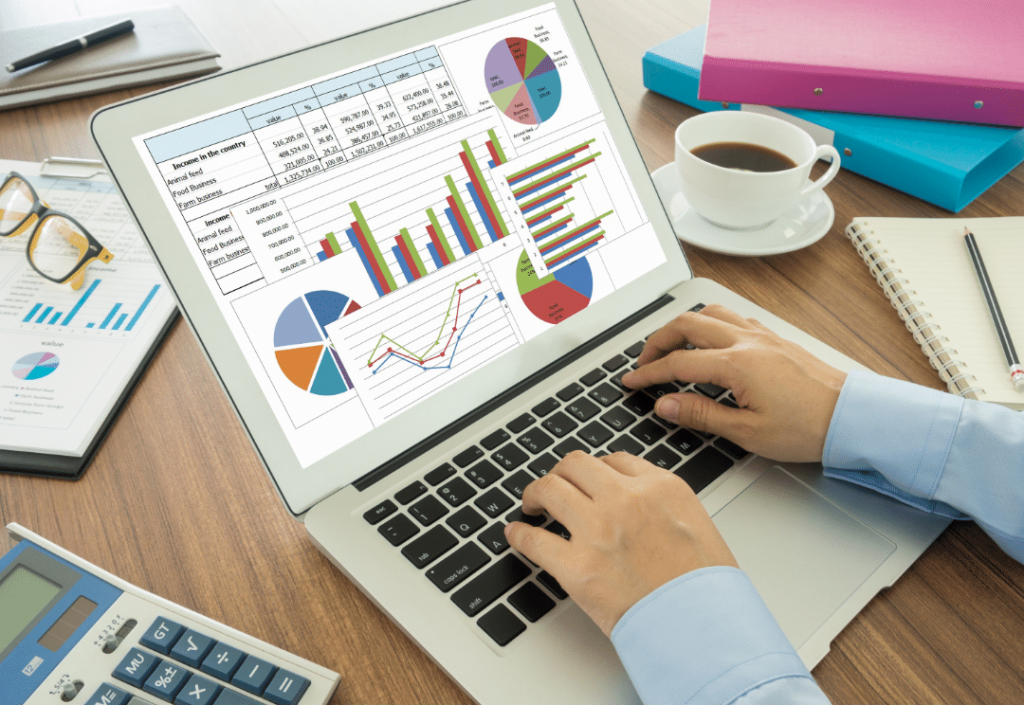
Content Credit:
- Article by Benedict Neo, published on builtin.com. Original article link.
Data is the most valuable resource of the 21st century. Across a breadth of industries, the world’s top companies are adapting their business models to collect and analyse more data. Governments and civil institutions are also following suit. Data analysis is an aspect of data science that is all about analysing data for different kinds of purposes. It involves inspecting, cleaning, transforming and modelling data to draw useful insights from it.
What are the different types of data analysis?
- Descriptive analysis
- Exploratory analysis
- Inferential analysis
- Predictive analysis
- Causal analysis
- Mechanistic analysis
With its multiple facets, methodologies and techniques, data analysis is used in a variety of fields, including — business, science and social science, among others. As businesses thrive under the influence of many technological advancements, data analysis plays a huge role in decision-making, providing a better, faster and more efficacious system that minimises risks and reduces human biases.
That said, there are different kinds of analysis catered for different goals.
1. Descriptive Analysis
The goal of descriptive analysis is to describe or summarise a set of data.
- Descriptive analysis is the very first analysis performed.
- It generates simple summaries of samples and measurements.
- It involves common, descriptive statistics like measures of central tendency, variability, frequency, and position.
2. Exploratory Analysis (EDA)
Exploratory analysis involves examining or exploring data and finding relationships between variables that were previously unknown.
- EDA helps you discover relationships between measures in your data, which do not evidence for the existence of the correlation, as denoted by the phrase, “Correlation doesn’t imply causation.”
- It’s useful for discovering new connections and forming hypotheses. It drives design planning and data collection.
3. Inferential Analysis
Inferential analysis involves using a small sample of data to infer information about a larger population of data.
- The goal of statistical modelling itself is all about using a small amount of information to extrapolate and generalise information to a larger group.
- Inferential analysis involves using estimated data that is representative of a population and gives a measure of uncertainty or standard deviation to your estimation.
- The accuracy of inference depends heavily on your sampling scheme. If the sample isn’t representative of the population, the generalisation will be inaccurate.
4. Predictive Analysis
Predictive analysis involves using historical or current data to find patterns and make predictions about the future.
- The accuracy of the predictions depends on the input variables.
- Accuracy also depends on the types of models. A linear model might work well in some cases, and in other cases it might not.
- Using a variable to predict another one doesn’t denote a causal relationship.
5. Causal Analysis
Causal analysis looks at the cause and effect of relationships between variables and is focused on finding the cause of a correlation.
- To find the cause, you have to question whether the observed correlations driving your conclusion are valid. Just looking at the surface data won’t help you discover the hidden mechanisms underlying the correlations.
- Causal analysis is applied in randomized studies focused on identifying causation.
- Causal analysis is the gold standard in data analysis and scientific studies where the cause of the phenomenon is to be extracted and singled out.
- Good data is hard to find and requires expensive research and studies. These studies are analysed in aggregate (multiple groups), and the observed relationships are just average effects (mean) of the whole population.
6. Mechanistic Analysis
Mechanistic analysis is used to understand exact changes in variables that lead to other changes in other variables.
- It’s applied in physical or engineering sciences, situations that require high precision and little room for error, the only noise in data is measurement error.
- It’s designed to understand a biological or behavioural process, the pathophysiology of a disease or the mechanism of action of an intervention.
…
Have you checked out yesterday’s blog yet?
Answering “Why do you want to work here?” At a Job Interview
(Disclaimer: The opinions expressed in the article mentioned above are those of the author(s). They do not purport to reflect the opinions or views of ICS Career GPS or its staff.)