Education & Career Trends: October 4, 2024
Curated by the Knowledge Team of ICS Career GPS
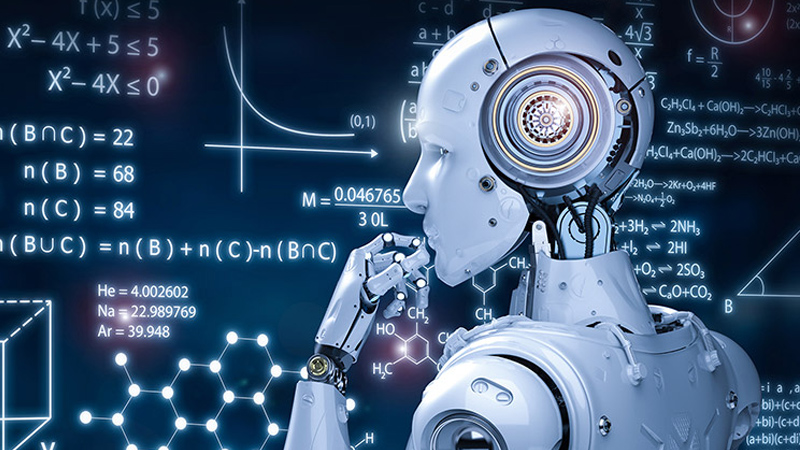
Article by Will Lockett, published on medium.com.
The current AI hype isn’t based on what today’s AI models can do, but on the belief that future versions will be revolutionary. Companies like Microsoft and JP Morgan are pouring billions into AI, not because the technology is great right now, but because they anticipate future models that could automate large parts of the workforce and rake in huge profits. However, this risky bet rests on shaky ground.
Recent research suggests AI might not improve as expected; in fact, it could be getting worse.
How AI Improvement Works
To understand this, it’s important to know how AI is supposed to get better. There are two main methods: scaling up and shaping up.
- Scaling up involves feeding more data into AI systems and increasing computational power. This has been the dominant strategy, but it’s hitting a wall. As the cost of data, infrastructure, and energy soars, this method becomes less feasible.
- Shaping up focuses on making existing AI models more efficient through fine-tuning based on human feedback. OpenAI’s recent “Strawberry” model, for example, uses a technique called “chain-of-thought prompting” to optimise performance without requiring more data. But even this approach has its limits.
AI is Actually Getting Worse
A study led by José Hernández-Orallo from the Polytechnic University of Valencia looked at large language models (LLMs) like those from OpenAI and Meta, and found that while they improved at complex tasks (like solving anagrams), they got worse at basic tasks, such as arithmetic. This pattern was echoed in other research, including a study from UC Berkeley that found similar declines in basic capabilities as newer AI models replaced older ones.
It’s not just chatbots that are struggling. Tesla’s Full-Self Driving (FSD) system has also shown signs of deteriorating performance in simple tasks as it becomes more “advanced.” These findings suggest that the AI industry’s current methods for advancing technology—whether through scaling up or shaping up—are fundamentally flawed.
The Reality: AI’s Limited Potential
One key takeaway from these studies is that improving AI in one area often means sacrificing performance in others. The hope was that methods like shaping up could allow AI to excel at both simple and complex tasks, but the results show otherwise. Instead, AI can only really specialise in certain areas, with broader applications proving elusive.
Even proposed solutions, like running multiple AIs in parallel to cover more ground, seem financially unfeasible given the current cost structure of AI development. The industry is already struggling to stay profitable, and the growing complexity only adds to the burden.
Conclusion: A Burst Bubble?
Hernández-Orallo’s study reveals a troubling reality: we are over-relying on AI, trusting it to do more than it’s capable of. The AI bubble, driven by dreams of future dominance, is on fragile ground. Not only are AI systems likely to fail at becoming the powerful, multi-functional tools envisioned by investors, but the cost of getting there could cripple the industry.
It’s time to reconsider how we approach AI development before this bubble bursts.
…
Have you checked out yesterday’s blog yet
7 Data Management Careers You Should Know About
(Disclaimer: The opinions expressed in the article mentioned above are those of the author(s). They do not purport to reflect the opinions or views of ICS Career GPS or its staff.)
One Reply to “AI Is Hitting Limitations It Simply Can’t Overcome”